Data Science vs. Data Analytics: Unraveling the Key Differences
In today's data-driven world, the terms "Data Science" and "Data Analytics" are often used interchangeably, leading to confusion for many. However, these are distinct fields with unique roles and purposes. In this article, we will explore the significant differences between data science and data analytics, shedding light on their specific functions, skills required, and the impact they have on businesses.
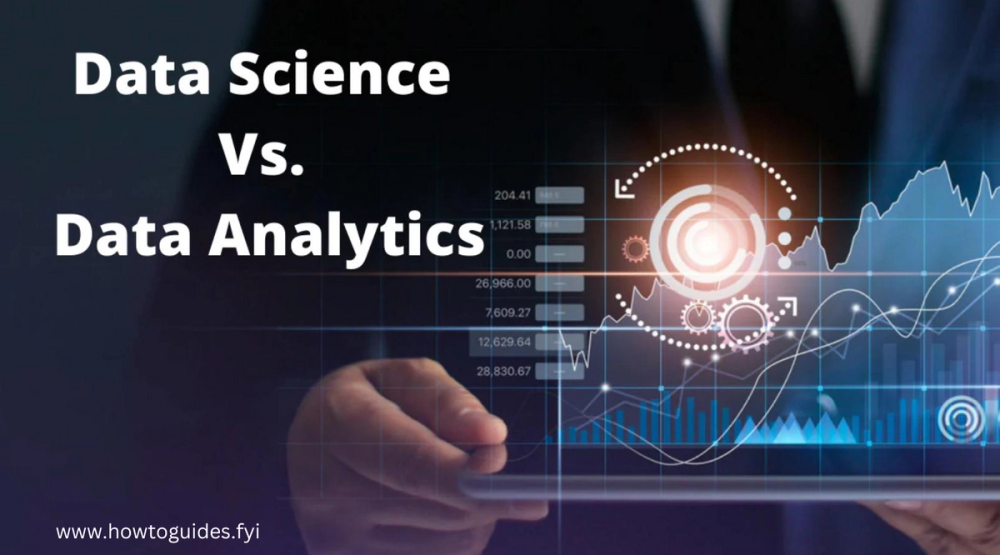
Introduction
In the era of big data, organizations are increasingly relying on data experts to extract valuable insights for informed decision-making. Two of the most prominent roles in this domain are Data Scientists and Data Analysts. While their objectives may overlap, their roles, skillsets, and approaches are fundamentally different.
Defining Data Science
What is Data Science?
Data Science is a multidisciplinary field that encompasses various techniques, algorithms, processes, and systems to extract meaningful insights and knowledge from structured and unstructured data. It involves a comprehensive understanding of statistics, programming, and domain knowledge to solve complex problems.
The Role of a Data Scientist
A Data Scientist is responsible for collecting, cleaning, and analyzing data to identify trends, patterns, and anomalies. They use advanced machine learning and data modeling techniques to build predictive models that can guide business strategies. Data Scientists play a crucial role in solving complex business problems and driving innovation.
Exploring Data Analytics
What is Data Analytics?
Data Analytics, on the other hand, focuses on examining data to draw conclusions and support decision-making. It primarily deals with historical data, aiming to answer specific questions and uncover insights that can improve business processes.
The Role of a Data Analyst
A Data Analyst's role is to gather data, clean and preprocess it, and perform statistical analysis. They create reports and dashboards to communicate their findings effectively. Data Analysts provide valuable information that aids in making data-driven decisions but usually work with predefined questions and datasets.
Data Science vs. Data Analytics: A Comparative Analysis
Now, let's dive into the critical distinctions between Data Science and Data Analytics.
Objectives and Goals
Data Science aims to solve complex problems and predict future outcomes by building sophisticated models. In contrast, Data Analytics focuses on examining historical data to answer specific questions and improve existing processes.
Skillset Required
Data Scientists require a deep understanding of mathematics, programming languages (like Python or R), and domain expertise. Data Analysts primarily need proficiency in data cleaning, visualization, and basic statistical analysis.
Tools and Technologies
Data Scientists often use complex tools for machine learning and data modeling, such as TensorFlow and scikit-learn. Data Analysts rely on tools like Excel, Tableau, or Power BI for data visualization and analysis.
Data Processing
Data Scientists work with both structured and unstructured data, often dealing with large datasets. Data Analysts mainly handle structured data that is already organized for analysis.
Decision-Making
Data Scientists contribute to strategic decision-making by providing insights into long-term trends and future predictions. Data Analysts support tactical decisions by providing immediate insights based on historical data.
Real-World Applications
5.1 Data Science Applications
Data Science is employed in various industries, including healthcare for disease prediction, finance for fraud detection, and e-commerce for recommendation systems.
Data Analytics Applications
Data Analytics finds applications in retail for sales forecasting, marketing for customer segmentation, and manufacturing for process optimization.
Which One to Choose?
Deciding between a career in Data Science or Data Analytics depends on your interests and career goals. If you enjoy diving deep into data, building predictive models, and solving complex problems, Data Science may be your path. On the other hand, if you prefer working with historical data to provide actionable insights and improve processes, Data Analytics could be your niche.
The Synergy Between Data Science and Data Analytics
In many organizations, Data Scientists and Data Analysts work collaboratively. Data Analysts provide the initial data insights that Data Scientists can further explore and model. This collaboration enhances decision-making processes.
Challenges in Both Fields
Both Data Science and Data Analytics face challenges related to data quality, privacy, and the need for constant skill updates. Staying updated with the latest tools and techniques is crucial in this ever-evolving field.
The Future of Data-Driven Careers
As businesses continue to embrace data-driven decision-making, the demand for skilled professionals in both Data Science and Data Analytics is expected to grow. This presents exciting career opportunities for those entering or already established in the field.
Conclusion
In summary, Data Science and Data Analytics are distinct yet complementary fields in the realm of data analysis. While Data Scientists focus on predictive modeling and complex problem-solving, Data Analysts provide actionable insights from historical data. Both roles are essential for organizations seeking to harness the power of data to drive success.
FAQs
What is the primary goal of data science?
The primary goal of data science is to extract meaningful insights and predictions from data to guide strategic decision-making.
Can you give an example of data analytics in action?
Certainly! Data analytics can be used in retail to analyze sales data and identify trends, helping businesses make informed decisions about inventory, pricing, and marketing strategies.
Do data scientists and data analysts work together?
Yes, they often collaborate in organizations. Data Analysts provide initial insights that Data Scientists can explore further with advanced techniques.
How do I decide between a career in data science or data analytics?
Consider your interests. If you enjoy predictive modeling and solving complex problems, data science may be a better fit. If you prefer working with historical data to provide insights, data analytics could be the right choice.
What skills are essential for success in both fields?
In both fields, proficiency in programming (Python or R), data cleaning, and data visualization is crucial. Data Scientists need additional skills in machine learning and data modeling, while Data Analysts benefit from strong statistical analysis abilities.
Related Guides
How to Download Cracked Version of Filmora: A Step-by-Step Guide
How to Write a Resignation Letter: A Comprehensive Guide
SEO-Optimized Guide: How to Create Do Follow Backlinks for Free
Discover the Top 10 Chat GPT Alternatives for Enhanced Conversations
How to Crack NEET in First Attempt: Strategies for Success
How to Crack UPSC in First Attempt: A Comprehensive Guide
How to Learn Canva: A Comprehensive Guide